Unlocking the Future: The Role of Data Labeling in Machine Learning for Home Services
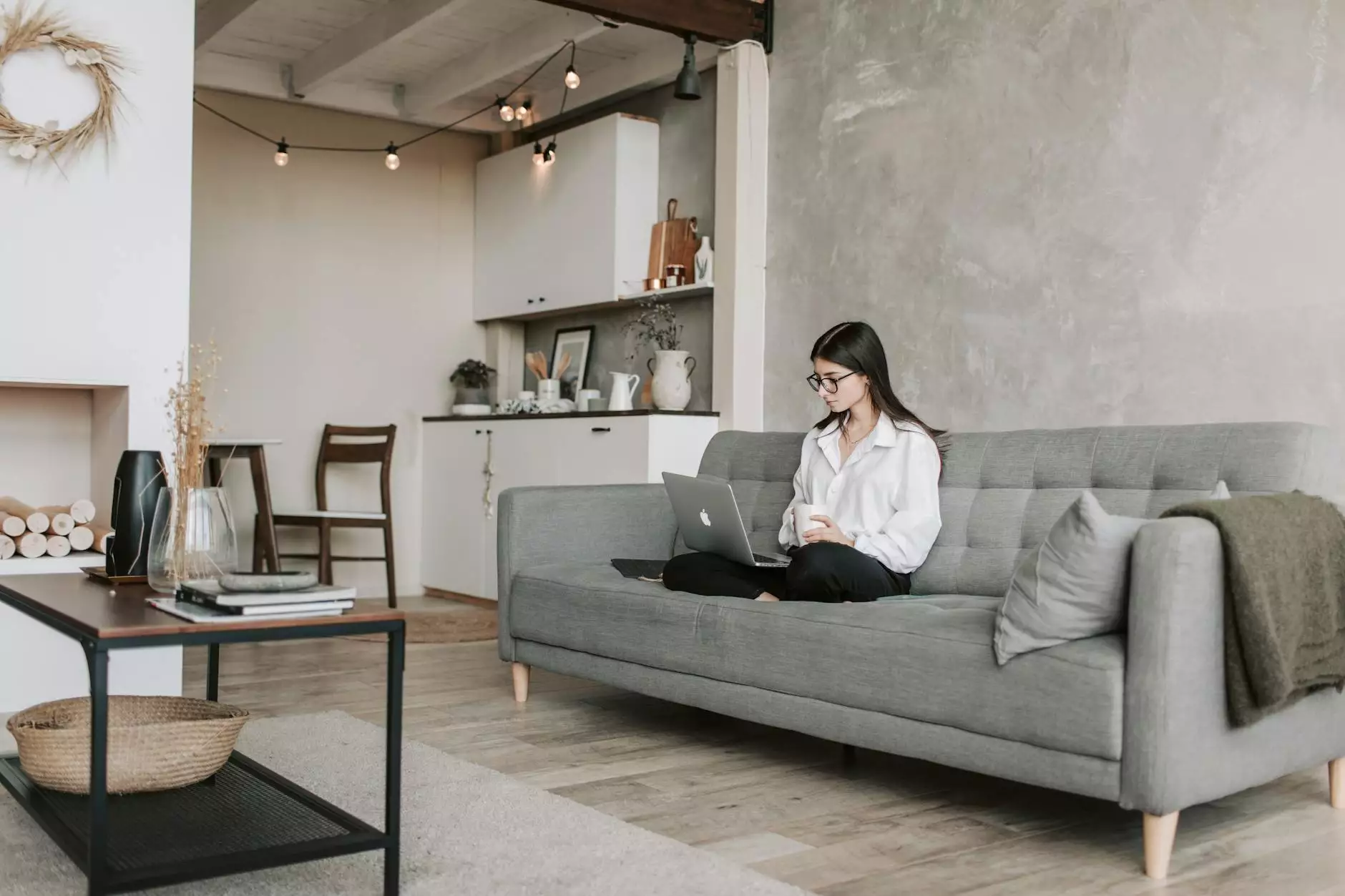
Data labeling is an essential component of machine learning that is often overlooked but holds incredible importance in various industries, including home services. As businesses strive to harness the power of artificial intelligence, understanding the intricacies of data labeling becomes crucial, particularly for sectors that rely on precise service delivery, such as keys and locksmiths.
What is Data Labeling?
Data labeling refers to the process of annotating or tagging data to provide context that machines can learn from. This process is vital for training machine learning models, as it transforms raw data into a structured format that AI algorithms can interpret and understand.
The Importance of Data Labeling in Machine Learning
Without data labeling, machine learning models would be lost in a sea of unorganized information. Properly labeled data instills a sense of meaning into the computational analysis, allowing models to make accurate predictions, automate processes, and derive insights. In the domain of home services, accurate data labeling can revolutionize the way services are offered, particularly in areas such as scheduling, customer service, and technical support.
Applications of Data Labeling in the Home Services Industry
The home services industry is vast and varied, encompassing numerous service providers. Here are some specific applications of data labeling for AI models in this industry:
1. Smart Scheduling Systems
Implementing machine learning for scheduling tasks in home services requires precise data from various sources. Data labeling helps AI understand factors such as:
- Customer preferences: Understanding which times customers prefer services.
- Availability: Data about technicians’ availability enhances scheduling efficiency.
- Traffic patterns: Understanding the best routes for service providers minimizes delays.
By labeling historical data related to past service requests, AI systems can predict when and where services are most needed, optimizing operations for locksmiths and other home service providers.
2. Enhanced Customer Interaction
Data labeling can drastically improve customer interaction within home services. By analyzing customer inquiries and feedback through natural language processing (NLP), businesses can:
- Identify common issues: Labeling customer conversations allows businesses to categorize issues.
- Automate responses: Train chatbots to respond accurately based on past interactions.
- Improve service quality: Analyze labeled data to continuously improve service delivery.
This level of customization not only enhances customer satisfaction but also helps businesses retain clients.
3. Predictive Maintenance
For service providers in the home services domain, such as locksmiths, preventive measures can save time and money. Through data labeling, machine learning algorithms can analyze previous maintenance records to predict when a lock might fail or need servicing. This predictive capability can result in:
- Reduced emergency calls: Anticipating issues minimizes emergency responses.
- Increased customer loyalty: Proactive services enhance customer retention.
- Efficient inventory management: Understanding usage patterns allows for better stock management.
Data Labeling Techniques for Machine Learning
To successfully integrate data labeling into machine learning processes, various techniques can be employed:
1. Manual Data Labeling
This traditional approach involves human annotators tagging the data. While it can be time-consuming, it ensures a high degree of accuracy and understanding, critical for complex tasks in home services.
2. Automated Data Labeling
With advancements in technology, automated data labeling is becoming more prevalent. Using machine learning algorithms, data can be labeled quickly, though it may require human audits to maintain accuracy.
3. Hybrid Data Labeling
This technique combines both manual and automated efforts to achieve a balance between speed and accuracy. By leveraging human expertise alongside machine efficiency, companies can label massive datasets effectively.
Benefits of Effective Data Labeling for Home Services
Investing in proper data labeling for machine learning applications in the home services sector offers numerous benefits:
- Increased Efficiency: Streamlined processes lead to quicker service delivery.
- Improved Accuracy: Accurately labeled data ensures that AI systems make reliable predictions.
- Enhanced Customer Experiences: Personalized and timely services increase customer satisfaction.
- Cost-Effective Operations: Predictive insights save costs associated with last-minute emergency services.
Challenges in Data Labeling for Machine Learning
1. Scale of Data
The sheer volume of data in home services can be overwhelming. Managing and labeling vast quantities of information requires a robust system and sufficient resources.
2. Consistency and Quality
Ensuring high-quality, consistent labeling across teams is critical. Inconsistencies can lead to faults in machine learning models, affecting decision-making processes.
3. Subjectivity in Labeling
Sometimes, data labeling can be subjective, especially when it involves human interpretation. Establishing clear guidelines and training for annotators is essential in maintaining objectivity.
Conclusion: The Future is Bright for Data Labeling in Home Services
The intersection of data labeling and machine learning presents immense opportunities for the home services industry. As businesses like KeyMakr adopt these technologies, they can enhance their operational efficiency, improve customer satisfaction, and stay ahead in a competitive market.
By prioritizing data labeling, home service providers, particularly those specializing in keys and locksmiths, can harness the full potential of machine learning, driving innovation and growth in their respective fields.
In conclusion, as we continue to explore and refine these technologies, the future of home services will undoubtedly be shaped by the profound impacts of effective data labeling in machine learning.
data labeling machine learning